Dr Yi Li
School of Mathematical and Physical Sciences
Lecturer
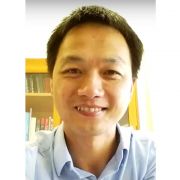
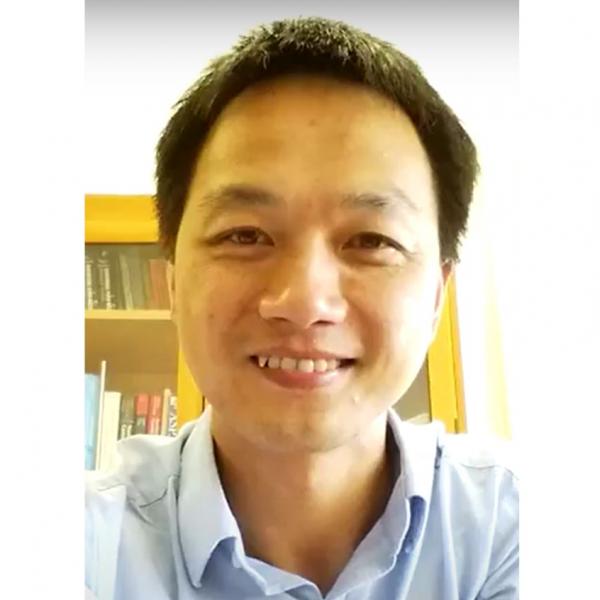
+44 114 222 3882
Full contact details
School of Mathematical and Physical Sciences (nee maths)
H14
Hicks Building
Hounsfield Road
Sheffield
S3 7RH
- Research interests
-
Dr Yi Li's main research interests are in the field of fluid mechanics, in particular turbulence. Topics include fluid flow optimisation, simulation and modelling, and the application of signal processing and database techniques in the study of data-intensive fluid mechanics problems.
Turbulent flows are characterised by chaotic or random eddies. They are ubiquitous in nature and industrial applications (for example the movement of the atmosphere, the waves in the ocean). Mathematically they are described by high dimensional chaotic nonlinear dynamical systems with a large number of variables. These variables describe the motion of eddies of very different sizes. Turbulent motion enhances mixing and heat exchange but its chaotic nature severely limits our ability, for example to make accurate weather prediction, or to plan pollution control, or to understand the formation of stars. It is a topic of tremendous importance. We rely heavily on numerical simulations as there are no general closed form solutions to the problem.
Research themes
Dr Yi Li’s research themes are shown below.
Downscaling
For multi-scale systems such a turbulent flow, the challenge very often is that it is too time-consuming to simulate all scales. A practical approach is to simulate only the large scales, which capture the main features of the flows, whereas the small scales are replaced by simpler models. This practice is called downscaling or subgrid-scale modelling. One fruitful idea in this line of research is to leverage the observation that turbulent flows are self-similar, very much like fractals. We have developed new downscaling approaches which exploit the self-similarity property in novel ways.
More about fractals on the Fractal Foundation website
Flow optimisation with data assimilation
Data assimilation refers to a suite of methods by which we synthesise model-based numerical simulations with available experimental or observational data to improve model predictions. The idea shares some similarities with the Bayesian approach in statistical modelling. A difference is perhaps the models here are derived from fundamental physical principles such as momentum and energy conservation; as such are mostly deterministic or strongly constrained.
Data assimilation has attracted more attention recently given increased data availability and computational capacity. We have been using the approach to optimise subgrid-scale models. How to combine the self-similarity properties of turbulent flows with the approach is a key question. We are also looking into how machine learning can be combined with data assimilation to enhance the predictive capability of the models.
Because turbulent flows are chaotic, turbulence simulation with data assimilation is also closely related to a fascinating subject called chaos synchronisation. Even though chaotic trajectories are unpredictable, it is possible to synchronise them so that two trajectories oscillate unpredictably, but in exactly the same way. We have looked into turbulence simulations through this lens. There are many interesting questions to be answered!
Stochastic modelling
A main feature of turbulent flows is the enhanced ability to spread and mix things up, which underpins many physical processes (for example cloud formation, dispersion of plastic particles in the oceans). To understand this aspect of turbulent flows, an approach is to investigate the stochastic motion of small particles or bubbles in a turbulent flow field. This approach is called stochastic modelling.
PhD Projects
There are opportunities for PhD projects in each of these themes. If you find them interesting, you can contact Dr Yi Li by email YiLi@shef.ac.uk or apply through the university PhD application portal.
- Publications
-
Journal articles
- Hot dip process of aluminum alloy based on nonlinear ultrasonic cavitation effect. Cailiao Gongcheng/Journal of Materials Engineering, 49(7), 133-140.
- Machine learning models for the secondary Bjerknes force between two insonated bubbles. Acta Mechanica Sinica, 37(1), 35-46.
- Modulation of the secondary Bjerknes force in multi-bubble systems. Ultrasonics Sonochemistry, 61. View this article in WRRO
- Small-scale reconstruction in three-dimensional Kolmogorov flows using four-dimensional variational data assimilation. Journal of Fluid Mechanics, 885. View this article in WRRO
- The secondary Bjerknes force between two oscillating bubbles in Kelvin-Voigt-type viscoelastic fluids driven by harmonic ultrasonic pressure. Ultrasonics Sonochemistry. View this article in WRRO
- Parallel chemistry acceleration algorithm with ISAT table-size control in the application of gaseous detonation. Shock Waves. View this article in WRRO
- The effects of ultrasonic treatments on the microstructure and mechanical properties of SAC0307 solder. Journal of Materials Processing Technology. View this article in WRRO
- Algorithm for transient growth of perturbations in channel Poiseuille flow. Applied Mathematics and Mechanics, 38(11), 1635-1650.
- Subgrid-scale stresses and scalar fluxes constructed by the multi-scale turnover Lagrangian map. Physics of Fluids, 29(4). View this article in WRRO
- Properties of a general quaternion-valued gradient operator and its applications to signal processing. Frontiers of Information Technology & Electronic Engineering, 17(2), 83-95. View this article in WRRO
- Adaptive Beamforming for Vector-Sensor Arrays Based on a Reweighted Zero-Attracting Quaternion-Valued LMS Algorithm. IEEE Transactions on Circuits and Systems II: Express Briefs, 63(3), 274-278. View this article in WRRO
- The evolution towards the rod-like axisymmetric structure for turbulent stress tensor. Physics of Fluids, 27(8). View this article in WRRO
- Constrained multi-scale turnover Lagrangian map for anisotropic synthetic turbulence: A priori tests. Physics of Fluids, 26(7), 075102-075102. View this article in WRRO
- Geometrical statistics of fluid deformation: Restricted Euler approximation and the effects of pressure. Comptes Rendus Physique, 13(9-10), 878-888. View this article in WRRO
- Small-scale intermittency and local anisotropy in turbulent mixing with rotation. J TURBUL, 12(38), 1-21. View this article in WRRO
- Geometrical statistics and vortex structures in helical and nonhelical turbulences. PHYS FLUIDS, 22(3). View this article in WRRO
- Lagrangian evolution of velocity increments in rotating turbulence: The effects of rotation on non-Gaussian statistics. Physica D: Nonlinear Phenomena, 239(20-22), 1948-1957. View this article in WRRO
- Matrix exponential-based closures for the turbulent subgrid-scale stress tensor. PHYS REV E, 79(1). View this article in WRRO
- A public turbulence database cluster and applications to study Lagrangian evolution of velocity increments in turbulence. Journal of Turbulence, 9, 1-29. View this article in WRRO
- Material deformation in a restricted Euler model for turbulent flows: analytic solution and numerical tests. Physics of Fluids, 19.
- Subgrid-scale modeling of helicity and energy dissipation in helical turbulence. Physical Review E (Statistical, Nonlinear, and Soft Matter Physics), 74.
- Intermittency trends and Lagrangian evolution of non-Gaussian statistics in turbulent flow and scalar transport. Journal of Fluid Mechanics, 558, 133-142.
- Origin of non-Gaussian statistics in hydrodynamic turbulence. Physical Review Letters, 95.
- Analysis of mean momentum flux in subgrid models of turbulence. Physics of Fluids, 16, 3483-3486.
- Study of Microstructure Scale and Regulation of Mechanical Properties of Al-6Si Hypoeutectic Alloy. Silicon.
- Synchronising large eddy simulations with direct numerical simulations via data assimilation. Physics of Fluids.
Conference proceedings papers
- Study of wind profile prediction with a combination of signal processing and computational fluid dynamics. 2017 IEEE International Symposium on Circuits and Systems (ISCAS), 28 May 2017 - 31 May 2017. View this article in WRRO
- A general quaternion-valued gradient operator and its applications to computational fluid dynamics and adaptive beamforming. 2014 19th International Conference on Digital Signal Processing, 20 August 2014 - 23 August 2014. View this article in WRRO
- A zero-attracting quaternion-valued least mean square algorithm for sparse system identification. 2014 9th International Symposium on Communication Systems, Networks & Digital Sign (CSNDSP), 23 July 2014 - 25 July 2014. View this article in WRRO
- Frequency-domain quaternion-valued adaptive filtering and its application to wind profile prediction. IEEE Region 10 Annual International Conference, Proceedings/TENCON
- Data exploration of turbulence simulations using a database cluster. SC '07 Proceedings of the 2007 ACM/IEEE conference on Supercomputing. Reno, Nevada, USA, 10 November 2007 - 16 November 2007.
Working papers
- View this article in WRRO
- Hot dip process of aluminum alloy based on nonlinear ultrasonic cavitation effect. Cailiao Gongcheng/Journal of Materials Engineering, 49(7), 133-140.
- Research group
- Teaching activities
-
MAS322 Operations Research MAS423 Advanced Operations Research MAS422 Magnetohydrodynamics MAS411 Topics in Advanced Fluid Mechanics