Dr Donghwan Shin
School of Computer Science
Lecturer in Testing
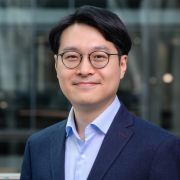
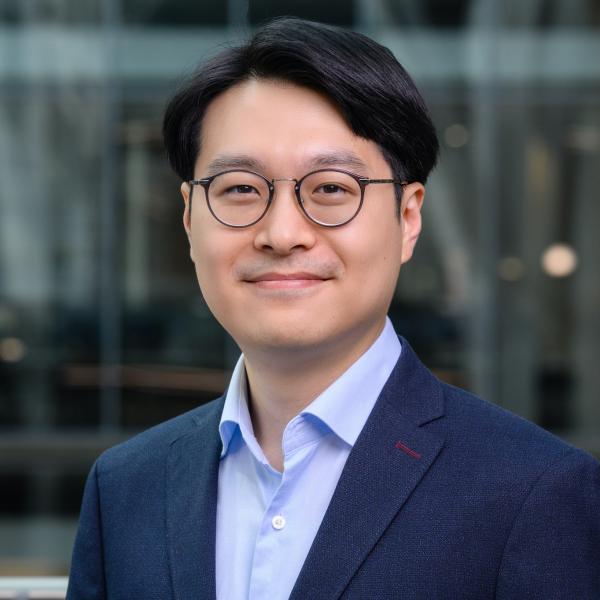
+44 114 222 1854
Full contact details
School of Computer Science
Regent Court (DCS)
211 Portobello
Sheffield
S1 4DP
- Profile
-
Dr Donghwan Shin is a Lecturer in Software Testing at the Department of Computer Science, University of Sheffield since 2022. He did his BS (2006-2010), MS (2010-2012), and PhD (2012-2018) at KAIST, South Korea. This is followed by four years as a research associate (2018-2020) and research scientist (2020-2022) at SnT, University of Luxembourg.
- Research interests
-
His research and teaching interests lie in mutation testing, testing for ML-enabled cyber-physical systems (e.g., ML-enabled automated driving systems), and log analysis (e.g., model inference and anomaly detection). He has published research papers at top venues such as ICSE, ICST, ISSTA, and MODELS and journals such as TSE, EMSE, and STVR.
- Publications
-
Journal articles
- Impact of log parsing on deep learning-based anomaly detection. Empirical Software Engineering, 29(6).
- Systematic Evaluation of Deep Learning Models for Log-based Failure Prediction. Empirical Software Engineering, 29(5).
- Summary of the Fourth International Workshop on Deep Learning for Testing and Testing for Deep Learning (DeepTest 2023). ACM SIGSOFT Software Engineering Notes, 48(4), 39-40.
- Identifying the Hazard Boundary of ML-enabled Autonomous Systems Using Cooperative Co-Evolutionary Search. IEEE Transactions on Software Engineering, 1-19.
- Correction to: Can Offline Testing of Deep Neural Networks Replace Their Online Testing?. Empirical Software Engineering, 27(6).
- PRINS : scalable model inference for component-based system logs. Empirical Software Engineering, 27(4).
- Can offline testing of deep neural networks replace their online testing?. Empirical Software Engineering, 26(5).
- Empirical evaluation of mutation-based test case prioritization techniques. Software Testing, Verification and Reliability, 29(1-2), e1695-e1695.
- A Theoretical and Empirical Study of Diversity-Aware Mutation Adequacy Criterion. IEEE Transactions on Software Engineering, 44(10), 914-931.
- An Applicability Study of Action-Benefit-Cost Model and Statistical Model Checking for System of Systems Goal Achievement Verification. KIISE Transactions on Computing Practices, 23(4), 256-261.
- A Case Study for Mutation-based Fault Localization for FBD Programs. KIISE Transactions on Computing Practices, 22(3), 145-150.
- Comprehensive analysis of FBD test coverage criteria using mutants. Software & Systems Modeling, 15(3), 631-645.
- Quality-Based Software Project Staffing and Scheduling with Project Deadline. Journal of KIISE, 42(7), 878-888.
- Human Resource Allocation in Software Project with Practical Considerations. International Journal of Software Engineering and Knowledge Engineering, 25(01), 5-26.
- Automated test case generation for FBD programs implementing reactor protection system software. Software Testing, Verification and Reliability, 24(8), 608-628.
- Rigorous Assessment of Model Inference Accuracy using Language Cardinality. ACM Transactions on Software Engineering and Methodology.
- Virtual Environment Model Generation for CPS Goal Verification using Imitation Learning. ACM Transactions on Embedded Computing Systems.
- An Extensible Modeling Method Supporting Ontology-based Scenario Specification and Domain-specific Extension. International Journal of Software Engineering and Knowledge Engineering.
Chapters
- Towards log slicing In Lambers L & Uchitel S (Ed.), Fundamental Approaches to Software Engineering (pp. 249-259). Springer Nature Switzerland
- A Theoretical Framework for Understanding the Relationship Between Log Parsing and Anomaly Detection, Runtime Verification (pp. 277-287). Springer International Publishing
- Empirical Evaluation on FBD Model-Based Test Coverage Criteria Using Mutation Analysis, Model Driven Engineering Languages and Systems (pp. 465-479). Springer Berlin Heidelberg
Conference proceedings papers
- Toward Automated Compliance Checking of Fund Activities Using Runtime Verification Techniques. Proceedings of the 1st IEEE/ACM Workshop on Software Engineering Challenges in Financial Firms, Vol. 18 (pp 19-20)
- Towards Simplification of Failure Scenarios for Machine Learning-Enabled Autonomous Systems. 2024 IEEE 24th International Conference on Software Quality, Reliability, and Security Companion (QRS-C) (pp 1089-1090), 1 July 2024 - 5 July 2024.
- Mutation-based Consistency Testing for Evaluating the Code Understanding Capability of LLMs. Proceedings of the IEEE/ACM 3rd International Conference on AI Engineering - Software Engineering for AI
- Tuning the Feedback Controller Gains is a Simple Way to Improve Autonomous Driving Performance. 2024 UKACC 14th International Conference on Control (CONTROL), 10 April 2024 - 12 April 2024.
- Many-Objective Reinforcement Learning for Online Testing of DNN-Enabled Systems. 2023 IEEE/ACM 45th International Conference on Software Engineering (ICSE), 14 May 2023 - 20 May 2023.
- Guidelines for assessing the accuracy of log message template identification techniques. Proceedings of the 44th International Conference on Software Engineering
- Efficient online testing for DNN-enabled systems using surrogate-assisted and many-objective optimization. Proceedings of the 44th International Conference on Software Engineering
- Automatic test suite generation for key-points detection DNNs using many-objective search (experience paper). Proceedings of the 30th ACM SIGSOFT International Symposium on Software Testing and Analysis
- Log-based slicing for system-level test cases. Proceedings of the 30th ACM SIGSOFT International Symposium on Software Testing and Analysis
- Digital Twins Are Not Monozygotic – Cross-Replicating ADAS Testing in Two Industry-Grade Automotive Simulators. 2021 14th IEEE Conference on Software Testing, Verification and Validation (ICST), 12 April 2021 - 16 April 2021.
- Comparing Offline and Online Testing of Deep Neural Networks: An Autonomous Car Case Study. 2020 IEEE 13th International Conference on Software Testing, Validation and Verification (ICST), 24 October 2020 - 28 October 2020.
- Formal Modeling and Verification of a Federated Byzantine Agreement Algorithm for Blockchain Platforms. 2019 IEEE International Workshop on Blockchain Oriented Software Engineering (IWBOSE), 24 February 2019 - 24 February 2019.
- Are mutation scores correlated with real fault detection?. Proceedings of the 40th International Conference on Software Engineering
- ABC
+. Proceedings of the 33rd Annual ACM Symposium on Applied Computing
- Mutation Analysis for System of Systems Policy Testing. 2017 IEEE/ACM Joint 5th International Workshop on Software Engineering for Systems-of-Systems and 11th Workshop on Distributed Software Development, Software Ecosystems and Systems-of-Systems (JSOS), 23 May 2017 - 23 May 2017.
- Diversity-Aware Mutation Adequacy Criterion for Improving Fault Detection Capability. 2016 IEEE Ninth International Conference on Software Testing, Verification and Validation Workshops (ICSTW), 11 April 2016 - 15 April 2016.
- A Theoretical Framework for Understanding Mutation-Based Testing Methods. 2016 IEEE International Conference on Software Testing, Verification and Validation (ICST), 11 April 2016 - 15 April 2016.
- Modeling and verification for different types of system of systems using PRISM. Proceedings of the 4th International Workshop on Software Engineering for Systems-of-Systems - SESoS '16, 14 May 2016 - 22 May 2016.
- A runtime verification framework for dynamically adaptive multi-agent systems. 2016 International Conference on Big Data and Smart Computing (BigComp), 18 January 2016 - 20 January 2016.
- Quality Based Software Project Staffing and Scheduling with Cost Bound. 2015 Asia-Pacific Software Engineering Conference (APSEC), 1 December 2015 - 4 December 2015.
- Efficient Testing of Self-Adaptive Behaviors in Collective Adaptive Systems. 2015 IEEE 39th Annual Computer Software and Applications Conference, 1 July 2015 - 5 July 2015.
Preprints
- Tuning the feedback controller gains is a simple way to improve autonomous driving performance, arXiv.
- Identifying the Hazard Boundary of ML-enabled Autonomous Systems Using Cooperative Co-Evolutionary Search, arXiv.
- Impact of log parsing on deep learning-based anomaly detection. Empirical Software Engineering, 29(6).
- Grants
-
SimpliFaiS: Simplification of Failure Scenarios for Machine Learning-enabled Autonomous Systems, UKRI, 01/01/2024 - 30/06/2026, £464,344, as PI