Dr Shaoxiong Sun
School of Computer Science
Lecturer in Pervasive Data Science
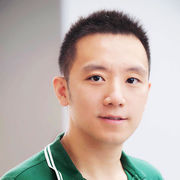
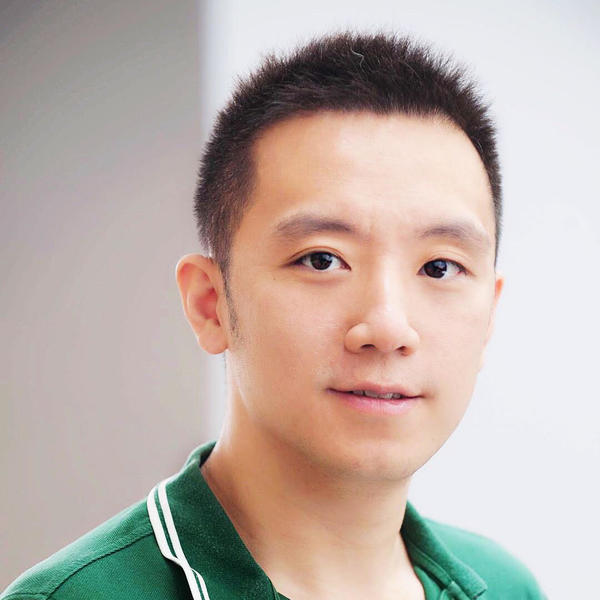
+44 114 215 7534
Full contact details
School of Computer Science
Regent Court (DCS)
211 Portobello
Sheffield
S1 4DP
- Profile
-
Dr. Shaoxiong Sun is a Lecturer in Pervasive Data Science at the Department of Computer Science, University of Sheffield. Previously, he was a Senior Research Associate in Data Science in Mobile Health at King’s College London. He was awarded a PhD from a joint program between Eindhoven University of Technology in the Netherlands and Philips Research, focused on physiological monitoring. He earned his BEng and MEng in Electrical Engineering from Harbin Institute of Technology and Dalian University of Technology, China, respectively.
Dr. Sun has over 30 peer-reviewed publications and 2 patents granted through the PCT system. He served on the technical program committees for IEEE CHASE 2022 and IEEE BSN 2023. He is also a guest editor for Frontiers in Signal Processing, Frontiers in Digital Health, and Algorithms.
Dr. Sun's research interests primarily revolve around physiological and behavioural monitoring, leveraging advanced signal processing and machine learning methodologies. In the realm of physiological monitoring, he focuses on estimating vital parameters such as blood pressure, heart rate, and respiration rate through the analysis of biomedical signals, such as photoplethysmography (PPG). In behavioural monitoring, his work centres on assessing mobility and social interaction patterns using wearable devices and smartphones. Dr. Sun has developed innovative technologies aimed at assisting individuals with a range of medical conditions, including depression, multiple sclerosis, epilepsy, ADHD, and COVID-19.
- Research interests
-
- Health data science
- Bedside and remote patient monitoring
- Wearable computing
- Cardiovascular monitoring
- Publications
-
Journal articles
- Physiological presentation and risk factors of long COVID in the UK using smartphones and wearable devices: a longitudinal, citizen science, case–control study. The Lancet Digital Health.
- Characterising the motion and cardiorespiratory interaction of preterm infants can improve the classification of their sleep state. Acta Paediatrica.
- Challenges in Using mHealth Data From Smartphones and Wearable Devices to Predict Depression Symptom Severity: Retrospective Analysis.. J Med Internet Res, 25, e45233.
- Remote Administration of ADHD-Sensitive Cognitive Tasks: A Pilot Study. Journal of Attention Disorders, 27(9), 1040-1050.
- Long-term participant retention and engagement patterns in an app and wearable-based multinational remote digital depression study.. NPJ Digit Med, 6(1), 25.
- The Feasibility of Implementing Remote Measurement Technologies in Psychological Treatment for Depression: Mixed Methods Study on Engagement.. JMIR Ment Health, 10, e42866.
- Systolic blood pressure estimation using ECG and PPG in patients undergoing surgery. Biomedical Signal Processing and Control, 79, 104040-104040.
- The utility of wearable devices in assessing ambulatory impairments of people with multiple sclerosis in free-living conditions. Computer Methods and Programs in Biomedicine, 227, 107204-107204.
- Temporal Evolution of Multiday, Epileptic Functional Networks Prior to Seizure Occurrence.. Biomedicines, 10(10).
- Associations Between Depression Symptom Severity and Daily-Life Gait Characteristics Derived From Long-Term Acceleration Signals in Real-World Settings: Retrospective Analysis.. JMIR Mhealth Uhealth, 10(10), e40667.
- Longitudinal Relationships Between Depressive Symptom Severity and Phone-Measured Mobility: Dynamic Structural Equation Modeling Study.. JMIR Ment Health, 9(3), e34898.
- The Association Between Home Stay and Symptom Severity in Major Depressive Disorder: Preliminary Findings From a Multicenter Observational Study Using Geolocation Data From Smartphones.. JMIR Mhealth Uhealth, 10(1), e28095.
- Fitbeat: COVID-19 estimation based on wristband heart rate using a contrastive convolutional auto-encoder. Pattern Recognition, 123, 108403-108403.
- Investigating the impact of COVID-19 lockdown on adults with a recent history of recurrent major depressive disorder: a multi-Centre study using remote measurement technology. BMC Psychiatry, 21(1).
- Predicting Depressive Symptom Severity Through Individuals' Nearby Bluetooth Device Count Data Collected by Mobile Phones: Preliminary Longitudinal Study.. JMIR Mhealth Uhealth, 9(7), e29840.
- Measuring the effect of Non-Pharmaceutical Interventions (NPIs) on mobility during the COVID-19 pandemic using global mobility data.. NPJ Digit Med, 4(1), 81.
- Relationship Between Major Depression Symptom Severity and Sleep Collected Using a Wristband Wearable Device: Multicenter Longitudinal Observational Study.. JMIR Mhealth Uhealth, 9(4), e24604.
- Using Smartphones and Wearable Devices to Monitor Behavioral Changes During COVID-19.. J Med Internet Res, 22(9), e19992.
- Finger and forehead photoplethysmography-derived pulse-pressure variation and the benefits of baseline correction. Journal of Clinical Monitoring and Computing, 33(1), 65-75.
- On algorithms for calculating arterial pulse pressure variation during major surgery. Physiological Measurement, 38(12), 2101-2121.
- Systolic blood pressure estimation using PPG and ECG during physical exercise. Physiological Measurement, 37(12), 2154-2169.
- Longitudinal Assessment of Seasonal Impacts and Depression Associations on Circadian Rhythm Using Multimodal Wearable Sensing: Retrospective Analysis. Journal of Medical Internet Research, 26, e55302-e55302.
Conference proceedings papers
- RADAR-base. Proceedings of the 2018 ACM International Joint Conference and 2018 International Symposium on Pervasive and Ubiquitous Computing and Wearable Computers
- Physiological presentation and risk factors of long COVID in the UK using smartphones and wearable devices: a longitudinal, citizen science, case–control study. The Lancet Digital Health.
- Research group