Dr Jill Johnson
School of Mathematical and Physical Sciences
Lecturer in Statistics
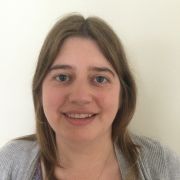
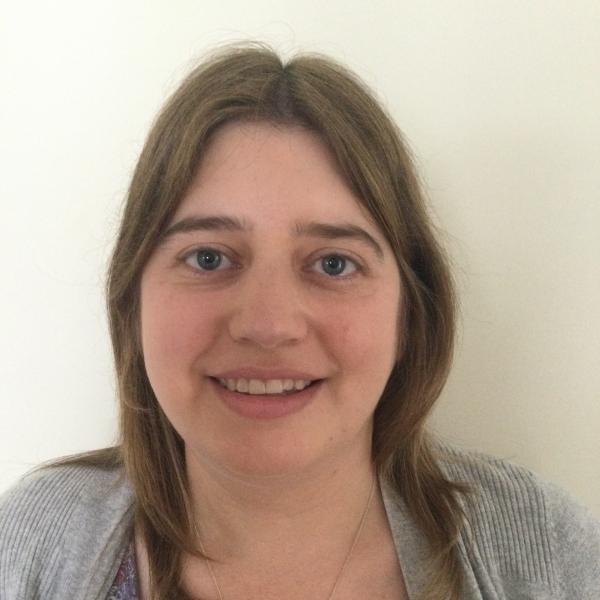
+44 114 222 3703
Full contact details
School of Mathematical and Physical Sciences
I11
Hicks Building
Hounsfield Road
Sheffield
S3 7RH
- Profile
-
Dr Johnson studied at Newcastle University, graduating in 2010 with a PhD in Statistics. Her thesis was titled ‘Modelling Dependence in Extreme Environmental Events’. Following this, she worked in the civil service as a research statistician at the government’s Food and Environment Research Agency, looking at uncertainty quantification and risk analysis for multiple applications including food safety and land use change.
In December 2012, Dr Johnson returned to academia as a research associate in the aerosol research group at the Institute for Climate and Atmospheric Science, University of Leeds, where her work focussed on the quantification and constraint of key uncertainties in complex models of the atmosphere and climate.
In August 2021 she joined the School of Mathematics and Statistics as a Lecturer in Statistics.
- Research interests
-
Uncertainty quantification for computer models; Surrogate modelling (emulation); Model-observation comparison (history matching).
My research interests are in the development and practical application of statistical methods to quantify, assess and then reduce uncertainty in large-scale complex models of real-world systems. To date, I have focussed on problems in environmental science and particularly in relation to the atmosphere and climate: I have worked with a range of models on different scales including the simulation of an individual cloud, to the simulation of a cloud field, to the simulation of the global distribution of aerosols in the atmosphere. My approaches include expert elicitation to inform parameter choices, the use of surrogate statistical models (Gaussian process emulation) to enable dense sampling over a complex model’s input uncertainties, sensitivity analysis to understand the driving sources of uncertainty and ‘history matching’ to reduce uncertainty via comparison to observations.
In my research, I aim to address the statistical challenges of model-observation comparison to constrain the effects of high-dimensional model parameter uncertainty when using diverse observations that have sparse spatial and temporal coverage, and to explore model uncertainty when a model output is non-stationary.
- Publications
-
Journal articles
- An Emulator of Stratocumulus Cloud Response to Two Cloud‐Controlling Factors Accounting for Internal Variability. Journal of Advances in Modeling Earth Systems, 16(9).
- Identifying climate model structural inconsistencies allows for tight constraint of aerosol radiative forcing. Atmospheric Chemistry and Physics, 23(15), 8749-8768.
- Simulating organic aerosol in Delhi with WRF-Chem using the volatility-basis-set approach: exploring model uncertainty with a Gaussian process emulator. Atmospheric Chemistry and Physics, 23(10), 5763-5782.
- Emulation of a chemical transport model to assess air quality under future emission scenarios for the southwest of Western Australia. Atmosphere, 13(12).
- Gaussian-process emulation for integrating data-driven aerosol-cloud physics from simulation, satellite, and ground-based data.
- Exploring a Stratocumulus-to-Cumulus Transition: A Perturbed Parameter Ensemble of Large-Eddy Simulations.
- Model emulation to understand the joint effects of ice-nucleating particles and secondary ice production on deep convective anvil cirrus. Atmospheric Chemistry and Physics, 21(23), 17315-17343.
- Unknown eruption source parameters cause large uncertainty in historical volcanic radiative forcing reconstructions. Journal of Geophysical Research: Atmospheres, 126(13). View this article in WRRO
- A perturbed parameter ensemble of HadGEM3-GC3.05 coupled model projections: part 1: selecting the parameter combinations. Climate Dynamics, 56(11-12), 3395-3436.
- A global model perturbed parameter ensemble study of secondary organic aerosol formation. Atmospheric Chemistry and Physics, 21(4), 2693-2723. View this article in WRRO
- Aerosol-cloud-climate cooling overestimated by ship-track data. Science, 371(6528), 485-489.
- Gaussian Process Modeling of Heterogeneity and Discontinuities Using Voronoi Tessellations. Technometrics, 63(1), 53-63. View this article in WRRO
- Effect of aerosol radiative forcing uncertainty on projected exceedance year of a 1.5 °C global temperature rise. Environmental Research Letters, 15(9). View this article in WRRO
- The value of remote marine aerosol measurements for constraining radiative forcing uncertainty. Atmospheric Chemistry and Physics, 20(16), 10063-10072. View this article in WRRO
- Robust observational constraint of uncertain aerosol processes and emissions in a climate model and the effect on aerosol radiative forcing. Atmospheric Chemistry and Physics, 20(15), 9491-9524. View this article in WRRO
- Constraining uncertainty in aerosol direct forcing. Geophysical Research Letters, 47(9). View this article in WRRO
- Environmental controls on tropical sea breeze convection and resulting aerosol redistribution. Journal of Geophysical Research: Atmospheres, 125(6). View this article in WRRO
- Comparing the impact of environmental conditions and microphysics on the forecast uncertainty of deep convective clouds and hail. Atmospheric Chemistry and Physics, 20(4), 2201-2219. View this article in WRRO
- Ensembles of global climate model variants designed for the quantification and constraint of uncertainty in aerosols and their radiative forcing. Journal of Advances in Modeling Earth Systems, 11(11), 3728-3754. View this article in WRRO
- Overview of the Antarctic Circumnavigation Expedition : study of preindustrial-like aerosols and their climate effects (ACE-SPACE). Bulletin of the American Meteorological Society, 100(11), 2260-2283. View this article in WRRO
- An emulator approach to stratocumulus susceptibility. Atmospheric Chemistry and Physics, 19(15), 10191-10203. View this article in WRRO
- Evaluation of global simulations of aerosol particle and cloud condensation nuclei number, with implications for cloud droplet formation.. Atmospheric Chemistry and Physics, 19(13), 8591-8617. View this article in WRRO
- Exploring how eruption source parameters affect volcanic radiative forcing using statistical emulation. Journal of Geophysical Research: Atmospheres, 124(2), 964-985. View this article in WRRO
- Using emulators to understand the sensitivity of deep convective clouds and hail to environmental conditions. Journal of Advances in Modeling Earth Systems, 10(12), 3103-3122. View this article in WRRO
- The importance of comprehensive parameter sampling and multiple observations for robust constraint of aerosol radiative forcing. Atmospheric Chemistry and Physics, 18(17), 13031-13053. View this article in WRRO
- Aerosol and physical atmosphere model parameters are both important sources of uncertainty in aerosol ERF. Atmospheric Chemistry and Physics, 18(13), 9975-10006. View this article in WRRO
- Climate models are uncertain, but we can do something about it. Eos, 99. View this article in WRRO
- Meteorological and land surface properties impacting sea breeze extent and aerosol distribution in a dry environment. Journal of Geophysical Research: Atmospheres, 123(1), 22-37. View this article in WRRO
- Global and regional trends in particulate air pollution and attributable health burden over the past 50 years. Environmental Research Letters, 12(10). View this article in WRRO
- The Global Aerosol Synthesis and Science Project (GASSP): measurements and modeling to reduce uncertainty. Bulletin of the American Meteorological Society, 98(9), 1857-1877. View this article in WRRO
- Aerosols in the pre-industrial atmosphere.. Current Climate Change Reports, 3(1), 1-15. View this article in WRRO
- New approaches to quantifying aerosol influence on the cloud radiative effect. Proceedings of the National Academy of Sciences, 113(21), 5812-5819. View this article in WRRO
- Evaluating uncertainty in convective cloud microphysics using statistical emulation. Journal of Advances in Modeling Earth Systems, 7(1), 162-187.
- Gaussian process emulation for second-order Monte Carlo simulations. Journal of Statistical Planning and Inference, 141(5), 1838-1848.
- The importance of comprehensive parameter sampling and multiple observations for robust constraint of aerosol radiative forcing.
- Aerosol and physical atmosphere model parameters are both important sources of uncertainty in aerosol ERF.
- Ensembles and uncertainty in climate change impacts. Frontiers in Environmental Science, 2.
Other
- Statistically optimising the input parameter space of a numerical ice sheet model to improve the model fit to observations of palaeo-ice flow direction .
Preprints
- An emulator of stratocumulus cloud response to two cloud-controlling factors accounting for natural variability., Authorea, Inc..
- Identifying climate model structural inconsistencies allows for tight constraint of aerosol radiative forcing, Copernicus GmbH.
- Identifying climate model structural inconsistencies allows for tight constraint of aerosol radiative forcing, Copernicus GmbH.
- Model emulation to understand the joint effects of ice-nucleating particles and secondary ice production on deep convective anvil cirrus, Copernicus GmbH.
- A global model perturbed parameter ensemble study of secondary organic aerosol formation, Copernicus GmbH.
- Ship-track-based assessments overestimate the cooling effect of anthropogenic aerosol, arXiv.
- Constraining uncertainty in aerosol direct forcing, Wiley.
- The value of remote marine aerosol measurements for constraining radiative forcing uncertainty, Copernicus GmbH.
- Robust observational constraint of uncertain aerosol processes and emissions in a climate model and the effect on aerosol radiative forcing, Copernicus GmbH.
- Comparing the impact of environmental conditions and microphysics on the forecast uncertainty of deep convective clouds and hail, Copernicus GmbH.
- An emulator approach to stratocumulus susceptibility, Copernicus GmbH.
- Evaluation of global simulations of aerosol particle number and cloud condensation nuclei, and implications for cloud droplet formation, Copernicus GmbH.
- Simulating organic aerosol in Delhi with WRF-Chem using the VBS approach: Exploring model uncertainty with a Gaussian Process emulator, Copernicus GmbH.
- An Emulator of Stratocumulus Cloud Response to Two Cloud‐Controlling Factors Accounting for Internal Variability. Journal of Advances in Modeling Earth Systems, 16(9).
- Research group
- Teaching activities
-
MAS113