Choosing a Hypothesis test
It can be difficult to know what analysis to perform on your dataset. This page is designed to help you.
Before you choose a test
Before you can choose how to analyse your data, there are three things you will need to understand:
1. What is a Hypothesis test?
Many statistical analyses are hypothesis tests. These are sometimes known as statistical tests. This is where a p-value is used to decide whether or not to reject a statement called the null Hypothesis.
2. Types of Data
Next, you need to be familiar with different types of data. Variables can be classified as continuous, categorical, ordinal etc. Usually one variable is an outcome/ dependent variable while you may have several predictor/independent variables.
3. Some introductory tests
Finally, you will need to know about some specific analyses, what they are designed to do and how to interpret the results.
We strongly recommend that you begin by trying to understand the following statistical processes in approximately this order. These are not the only statistical techniques available but without an understanding of these processes, it’s usually difficult to understand other analyses.
- T-tests (paired and unpaired)
- Chi-Squared tests
- ANOVA (one-way, repeated measures and two-way)
- Simple Linear Regression
- Multiple Regression
Choosing a test
You can use your knowledge of the topics above to begin identifying a test that will help you answer your research question. Ideally, we make a plan for the analysis before the data are collected.
Once you have decided on the test you would like to use, you need to check the assumptions. These are conditions which your data will need to meet if the hypothesis test is going to work. Tests which require the data to meet assumptions are called parametric tests. If you find that your data don’t meet the assumptions for the test you would like to perform, you may find there is a non-parametric test you can use instead.
What Test Tables
The charts below are designed to help you identify when to use some tests and their non-parametric alternatives.
Please note, these tables only show the most common tests.
Comparing Means (continuous variables)
Paired or unpaired observations |
Test |
Assumptions not met |
|
---|---|---|---|
1 group |
Comparing the mean to a fixed number |
One-sample t-test |
One-sample Wilcoxon signed-rank test |
2 groups |
Unpaired |
Independent samples t-test |
Mann-Whitney U test |
2 groups | Paired | Paired t-test | Wilcoxon Signed rank test |
3+ groups |
Independent groups |
One-way ANOVA |
Kruskal-Wallis test |
3+ groups | Repeated measurements | Repeated Measures ANOVA | Friedman test |
2 grouping variables |
Both grouping variables categorical, neither with repeated measures |
Two-way ANOVA |
No simple answer |
2 grouping variables | Both grouping variables categorical, one with repeated measures | Mixed ANOVA | Friedman test |
Comparing Count data (categorical variables)
Paired or unpaired observations |
Test |
Assumptions not met |
---|---|---|
Unpaired |
Chi-squared test |
Fisher’s exact test |
Paired |
McNemar’s test |
No simple answer |
Investigating Relationships
Variables |
Dependent |
Independent |
Analysis |
---|---|---|---|
2 variables |
continuous |
continuous |
Simple Linear Regression |
2 or more |
continuous |
Two or more, any kind |
Multiple Linear Regression |
2 or more |
binary |
One or more, any kind |
Binary Logistic Regression |
2 or more |
categorical |
One or more, any kind |
Logistic Regression |
Links
These tables are our way of thinking through the different analyses available. There are many alternatives. Here are some links to websites we think are helpful:
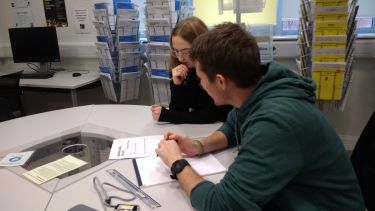
Book a 1:1 appointment or workshop
Would you like to explore a maths or stats topic in greater depth? Why not book a 1:1 with an advisor or a workshop (current students only).