Control and Systems Engineering
Control and Systems Engineering research at Sheffield has a proud history dating back to the 1960s. Our work spans both theory and applications, with a focus on developing methods that support multi-disciplinary research and address emerging engineering and societal challenges.
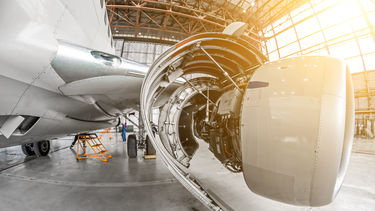
We collaborate with industry, and other research themes within the School and across Sheffield, as well as universities worldwide. We host the Rolls-Royce University Technology Centre in Control, Monitoring and Systems Engineering, where we drive innovations in control systems, health monitoring, and diagnostics for aerospace and other high-performance engineering applications.
Control
Model Predictive Control (MPC) and Dynamic Optimization
We have considerable expertise in MPC as a powerful tool for providing optimal control in dynamic environments, ensuring real-time performance and adaptability. Our work includes developing predictive and optimization-based control frameworks, such as robust, adaptive, and learning-based MPC, and real-time numerical dynamic optimization methods, with focus on achieving certificates of safety and stability while maintaining manageable computational complexity. Our applications span energy systems, aerospace, autonomous vehicles, and large-scale infrastructure.
Networked, Distributed, and Multi-Agent Systems
Networked, distributed, and multi-agent systems, such as transportation networks, power grids, and intelligent infrastructure are integral to modern engineering and technology. Our research develops theory and tools for the identification, analysis and design of these complex networks, addressing challenges posed by high-dimensional dynamics, time delays, dynamic topologies, and communication interruptions, and cyberattacks.
Robust and Nonlinear Control
We focus on developing control techniques that ensure system performance and stability under uncertainty and nonlinearity. Our expertise includes robust control design and analysis methods for certifying the safe operation of complex systems operating in uncertain environments and nonlinear identification methods including NARMAX and LPV models. Our applications range from enhancing infrastructure resilience to managing advanced engineering systems such as gas turbine engines, electric power plants, and battery energy storage systems.
Model Identification and Learning-based Control
We cover both traditional system identification and emerging data-driven methods for building dynamic models of systems. Our techniques include identifying and controlling complex systems based on spatiotemporal data and employing learning-based approaches where adaptive models continuously improve. Our applications span from physical systems such as batteries, power plants, and autonomous vehicles to virtual systems such as neural networks and AI-based models where learning plays a crucial role.
Applications of Control (including Control Education)
We apply our expertise in control theory to a range of practical domains, and traditional and emerging applications, including battery modelling and optimization, power systems and smart grids, urban mobility, and transportation networks, and societal systems. Our focus also extends to control education, where we investigate and develop novel problem-based learning techniques, technological tools, and curricula to enhance learning of control theory and engineering and promote its role in solving the many current and future engineering and societal challenges.
Dynamical Systems and Systems Engineering
Complex System Modelling, Analyses, and Designs: Theories, Methods, and Applications
One focus of this research is on the identification of the models of complex nonlinear systems that can readily be used to reveal the underlying dynamics rather than just fit the data, and provide insight into or predict fundamental physical properties of the systems of interest. This is concerned with the further development of the well-known NARMAX methodology which was uniquely created at Sheffield and has been widely applied in various science and engineering areas.
Another focus is on the theories and methods for the analysis and design of nonlinear dynamic systems in the frequency domain, which were again initiated at Sheffield and involve the derivation of a range of new concepts and approaches including. e.g, Nonlinear Output Frequency Response Functions (NOFRFs). The NOFRFs is a novel extension of the well-known linear system concept of Frequency Response Function (FRF) to the nonlinear case, has been adopted by many researchers to resolve different science and engineering problems and has recently, for the first time, enabled the real-time analysis of nonlinear engineering systems in the frequency domain.
In collaboration with academic and industrial partners in a wide range of disciplinary fields, the outcomes of these theoretical and methodological developments have been effectively applied by the group to address various real-world challenges including, e.g, dynamic designs of material systems, aerospace structure optimization, advanced manufacturing system operational condition monitoring, medical diagnosis, structural system vibration control, and infrastructure (buildings, bridges, wind turbines, etc) health monitoring and fault diagnosis.
The methods and tools developed by the group have been successfully used to develop the Sheffield online space weather forecasting tools, provide forecasts of geomagnetic indices and the electron radiation in the radiation belts, which have been shown to provide the most accurate two-day-ahead forecast available of the electron flux at geostationary orbit and will be implemented at the UK Met Office. Forecasting the evolution of these fluxes enables mitigation of their effects on spacecraft. The methods developed by this group have been applied to seasonal weather and climate recently; the research results and findings obtained to date are highly promising.
The group is also carrying out research into medical, neurosciences, biosciences and environmental systems, using data-driven modelling and interpretable machine learning and AI techniques to derive explainable models from measured datasets.
Complex, Spatio-Temporal Systems
Many biological, chemical and physical systems and other complex systems (e.g. weather and climate, space weather and environment, mitochondrial dynamics, tumour growth and other diseases) involve variables that depend on space and time. Such systems, which often operate away from equilibrium, exhibit complex emergent behaviour such as pattern formation, self-organisation, turbulence, spatio-temporal chaos, distinct populations of elements that can interact via sink and source terms in their conservation equations etc.
The goal of such research is to use measured data and extract informative structures, reconstruct the underlying spatio-temporal dynamics that govern these systems as well as analyse, understand and forecast their emerging properties. The realisation, identification, prediction, analysis and control of this class of systems are being studied at the theoretical level, to develop practical methodologies and tools that can help address big research challenges in energy, environment, life sciences, and healthcare etc.
The tools developed as part of this research have been successfully applied to study iceberg calving patterns, probabilistic predictions of seasonal weather and climate change, causes and impacts of atmospheric changes, identification of the drivers of space weather, MHD waves and coherent plasma structures in the solar atmosphere, control of crystal growth, fluid flows and wildfires.
The group is also carrying out research on the geospace plasma processes, and the interactions of plasmas with high-energy beams of particles, the multiscale modelling of musculoskeletal systems in biomedical system engineering, the cellular response to heat shock and hypoxia, and the modelling and simulation of mitochondrial dynamics in response to energy stress.
Systems Engineering of Control and Monitoring Systems
Systems Engineering is actively applied within the group to manage the complexity that emerges from non-linear cyber-physical systems. Formalising the systems thinking embedded within the school, our Systems Engineering expertise is applied in partnership with our research partners across the industry. Our research underpins programmes to consider not only the sense-to-act feedback loop but beyond to capture, assess and build/adapt systems to meet multi-party techno-social goals. In partnership, we develop and integrate sensor systems, signal processing and control algorithms within a systems framework to deliver against elicited requirements. These research applications underpin our goal in Systems Engineering teaching that will deliver engineers adept at problem-solving at a range of systems and scales.
Group members have developed intelligent detection, diagnosis and prognosis methods using machine learning and AI techniques, and applied them to complex industrial plants and machinery such as wind power turbines and aerospace propulsion systems.