What deep nets can & can’t do with language and why
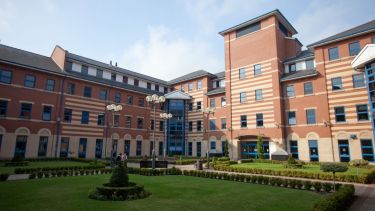
Event details
Description
Abstract: Current deep neural net (dNN) systems have been claimed to extract a near human-like knowledge of language (KoL)— and use it. But is this really so? In this talk we will make these points:
1. The most advanced dNNs, e.g., ChatGPT4 and beyond, fail on some of the oldest classical tests of KoL, as well as other human cognitive abilities, with implications for their use in “real world” settings. It is not clear how to “fix” these “holes” in the dNN systems, because what the dNNs acquire internally need not correspond to what humans acquire.
2. We can precisely describe what dNNs do in geometric terms, demonstrating that they can only in effect only linearly interpolate between regions they have been trained on, rather than truly extrapolate as human children do.
Joint work with Robert Ajemian, Karthik Srinivasan, and Sabrina Drammis
About the Speaker: Professor Berwick and his research group investigate computation and cognition, including computational models of language acquisition, language processing, and language change, within the context of machine learning, modern grammatical theory, and mathematical models of dynamical systems. A second line of inquiry is probing the biological and evolutionary underpinnings of human language, including models of language change as well as its biologically-grounded evolutionary origins, in particular, in birdsong.Professor Berwick has been the recipient of a John Simon Guggenheim Memorial Award as well as the MIT Edgerton Faculty Achievement Award, MIT’s highest honor for junior faculty. He has also received an NSF Presidential Young Investigator Award. He helped found and run MIT’s Center for Biological and Computational Learning for more than 15 years
Location
53.381049514166, -1.4801965649731
When focused, use the arrow keys to pain, and the + and - keys to zoom in/out.