Seminar with Bioinformatics Institute's Dr Hwee Kuan Lee
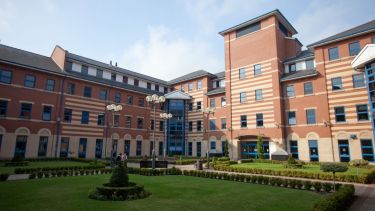
Event details
Description
Abstract: Fully supervised Deep Learning methods are very effective in providing high performance. However, this approach has two major drawbacks. Firstly fully supervised methods require tedious expert annotations. Secondly, the AI trained on expert labels cannot surpass the standard of the expert and therefore AI performance degrades as the labels become noisy. We proposed an indirect way of training our AI models using a weakly supervised, multi-instance, multi-task learning paradigm that avoids detailed annotations. With this indirect way of training our AI, we demonstrated that our learning paradigm can elucidate instance-level features very well. This work also provides mathematical guarantees on some properties of our weakly supervised method.
Biography: Hwee Kuan Lee’s current research involves developing Artificial Intelligence (AI) research for clinical and biological applications. His laboratory focuses on diverse research activities, including more basic AI-centric research as well as AI applications. Clinical application areas include diagnostics in cancers, spatial omics, cardiology, dermatology and interventional radiology. In the area of biology, Hwee Kuan’s laboratory works on the development of AI in protein science and drug discovery. Hwee Kuan’s primary appointment is as the Deputy Director for Training and Talent development at the Bioinformatics Institute. He also holds multiple adjunct and joint appointments in the local universities and other research institutions.
Location
53.3725184, -1.4876672
When focused, use the arrow keys to pain, and the + and - keys to zoom in/out.