Benjamin Scellier's talk on Equilibium Backpropagation
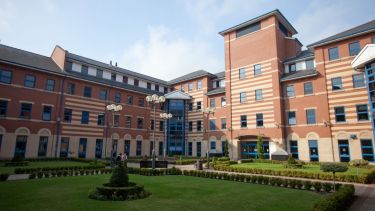
Event details
Description
Speaker
Benjamin Scellier, Principal Research Scientist at Rain Neuromorphics
Abstract
We present a mathematical framework of learning called "equilibrium propagation" (EP). The EP framework is compatible with gradient-descent optimization -- the workhorse of deep learning -- but in EP, inference and gradient computation are achieved using the same physical laws, and the learning rule for each weight (trainable parameter) is local, thus opening a path for energy efficient deep learning. We show that EP can be used to train electrical circuits composed of voltage sources, variable resistors and diodes – a class of networks that we dub "deep resistive networks" (DRNs). We show that DRNs are universal function approximators: they can implement or approximate arbitrary input-output functions. We then present a fast algorithm to simulate DRNs (on classical computers) as well as simulations of DRNs trained by EP on MNIST. We argue that DRNs are closely related to deep Hopfield networks (DHNs), and we present simulations of DHN trained by EP on CIFAR10, CIFAR100 and ImageNet 32x32. Altogether, we contend that DRNs and EP can guide the development of efficient processors for AI.
Location
53.381097551434, -1.4799640168528
When focused, use the arrow keys to pain, and the + and - keys to zoom in/out.