Vacancies
We regularly have exciting opportunities for researchers to join our team.
If there are no positions currently listed you may wish to subscribe to our monthly newsletter and stay informed of any opportunities in the future.
Current vacancies
Research Assistant in AI for Intelligent Sports Systems
Closing date: 28 April 2024
Employer: The University of Sheffield (Department of Automatic Control and Systems Engineering)
Location: Sheffield
Salary: Grade 6.1 £30,487 per annum
Vacancy number: UOS040699
Job Description
Are you passionate about sports, AI, and entrepreneurship?
We're seeking a Research Assistant to revolutionise sports training using Artificial Intelligence and robotics. At the forefront of research and innovation in sports technology, this project holds immense potential for commercialization and is currently in Stage 2 of the Commercialization Journey at the University of Sheffield.
You will join Dr Lin Cao’s world-leading robotics lab in the Department of Automatic Control and Systems Engineering at the University of Sheffield. You will hold a Bachelor’s or Master’s degree in Computer Science, Electrical Engineering, Robotics, or a related field (fresh graduates are welcome!) and have strong skills in AI, programming, problem-solving, and communication. You will have the unique opportunity to hone and apply your AI and programming skills in an innovative project strongly supported by academia and the sports industry and meanwhile be deeply involved in the commercial development of the project as a co-founder.
We’re one of the best not-for-profit organisations to work for in the UK. The University’s Total Reward Package includes a competitive salary, a generous Pension Scheme and annual leave entitlement, as well as access to a range of learning and development courses to support your personal and professional development.
We build teams of people from different heritages and lifestyles from across the world, whose talent and contributions complement each other to greatest effect. We believe diversity in all its forms delivers greater impact through research, teaching and student experience.
How to apply
To apply visit our job pages (https://www.sheffield.ac.uk/jobs) and search for vacancy number: UOS040699.
Contact details
For informal enquiries about this job contact
Dr Lin Cao, Lecturer: l.cao@sheffield.ac.uk
Dr Ehsan Alborzi, Senior Fellow: e.alborzi@sheffield.ac.uk
Research Associate in Soft Robotic Implantable Technologies for Short Bowel Syndrome
Closing date: 30 April 2024
Employer: The University of Sheffield (Department of Automatic Control and Systems Engineering)
Location: Sheffield
Salary: Grade 7: £37,099 per annum
Vacancy number: UOS040569
Job Description
We have an exciting opportunity in the University of Sheffield’s Department of Automatic Control and Systems Engineering, in the Sheffield Biomedical Robotics Laboratory for someone with a passion for the fields of soft robotics, and medical and surgical robotics and a keen interest to see these technologies translate to the clinic.
The post provides a rare opportunity to contribute to research and development of transformative surgical techniques for the repair and reconstruction of soft tissues. This project unifies three lines of research: robotics, soft functional materials and mechanical-driven therapy.
You will contribute with publications, developing preclinical-proof devices in collaboration with stakeholders, assist the surgical team with the engineering aspects of the device preclinical testing and lead preliminary activities for the device commercialisation. This post is funded by MRC, with co-investigators from Sheffield Children’s Hospital, Sheffield Royal Hallamshire Hospital, and our industrial partners. This project is part of an academically led commercial opportunity with the vision to develop a cure for Short Bowel Syndrome. The team is part of and supported by the Insigneo Institute, an organisation driving innovative research at the interface of healthcare, engineering, and science to transform the future of healthcare technology and is supported by a dedicated Commercialisation Manager.
You should hold a PhD (or be close to completion) in any relevant area of robotics, soft robotics, medical robotics (or have equivalent experience) and should have a strong knowledge of experimental implementation of soft-matter fabrication or/and control and be interested in taking a technical lead in the translation of the technology. You will join an internationally renowned research group in biomedical robotics, and will contribute to the reputation of ACSE as the leading department in systems and control.
The principal investigator and line manager for this role is Dr. Dana Damian. Her research has received international awards, e.g., Best Paper Award in Medical Robotics at ICRA 2020, and extensive media coverage, including Forbes, The Economist, New Scientist, The Telegraph, USA Today, National Geographic. More information about the lab:
Website: https://sites.google.com/site/danadamian/
Youtube: https://youtube.com/playlist?list=PLk5c3UK4_AtgCLdj1CsKsDcB1tSnUJJeU
We’re one of the best not-for-profit organisations to work for in the UK. We build teams of people from different heritages and lifestyles from across the world, whose talent and contributions complement each other to greatest effect. We believe diversity in all its forms delivers greater impact through research, teaching and student experience.
How to apply
To apply visit our job pages (https://www.sheffield.ac.uk/jobs) and search for vacancy number: UOS040569.
Contact details
For informal enquiries about this job contact Renata Goddard and Lewis Bolam on acseresearchrecruitment@sheffield.ac.uk
PhD Opportunity: Biomechanics of internal fixation methods for ankle fusion
Closing date: 18 June 2024
Employer: The University of Sheffield (Department of Mechanical Engineering)
Location: Sheffield
About the Project
Ankle fusion is a common treatment for advanced ankle arthritis to relieve pain and improve functional outcomes. However, there is a reported non-union rate of 5-37%, requiring further surgery or an ankle replacement. Moreover, there are several options for internal fixation, with no consensus on the number of screws, orientations, and placement in ankle fusion surgery.
This project aims to develop validated computational models of the foot and ankle, and investigate the biomechanical parameters impacting successful bone healing while reducing the risk of fixation failure.
The project will involve parametric analysis of participants’ data, finite element analysis and mechanical testing. Hence, the candidate will be consulting with collaborators in orthopaedic surgery, bioengineering, mechanical engineering and the Insigneo Institute. The candidate will gain a unique opportunity to carry out research in an exciting interdisciplinary project to answer important clinical questions.
Requirements
We are looking for applicants who are eager to develop new skills and apply their existing knowledge. You should be excited to learn and develop new technology, be passionate about the subject and look to be creative in your work. In addition, you should:
- Hold (or will receive this year) a master's degree (or equivalent, e.g. MEng) at merit/distinction level, or a first class/ upper second (2:1) class honours degree in biomedical engineering, mechanical engineering, applied maths or a related field.
- Proficiency in finite element analysis software (e.g., Abaqus, Ansys etc) and programming languages such as Python, MATLAB etc.
- Strong analytical and problem-solving skills.
- Good interpersonal and organisational skills, and the ability to work as a team player.
Interested candidates are strongly encouraged to contact Dr Vee San Cheong (v.cheong@sheffield.ac.uk), to discuss your interest in and suitability for the project prior to submitting your application.
Funding Notes
This studentship includes a fee bursary to cover the UK (home) rate, and an annual tax-free maintenance stipend at the standard UK Research rate (£19,237 in 2024-25) for up to 3.5 years. Overseas applicants will need to secure additional funding to cover the difference in fees, or with their own funds which is approximately £22,884 per year. Overseas applicants should ensure they can pay the difference in fees prior to applying or sending any enquiries.
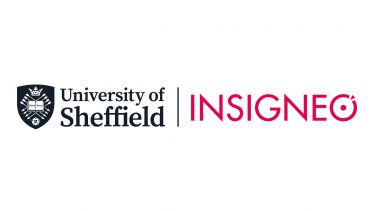