Insigneo Seminar: Predicting atrial fibrillation treatment outcomes through computational modelling
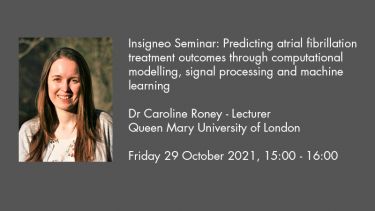
Event details
Description
Insigneo Seminar: Predicting atrial fibrillation treatment outcomes through computational modelling, signal processing and machine learning
We are delighted to announce our next Insigneo Seminar will be a hybrid event with Dr Caroline Roney, a lecturer in Computational Medicine and a Medical Research Council Skills Development Fellow in the School of Engineering and Materials Science, Queen Mary University of London, presenting in person on ‘Predicting atrial fibrillation treatment outcomes through computational modelling, signal processing and machine learning’ on Friday 29 October 2021. Insigneo Members please check your calendar invitations for online joining details. To request an invitation please contact info@insigneo.org. In Person venue: Alfred Denny Conference Room, Alfred Denny Building.
Short bio:
Dr Caroline Roney is a lecturer in Computational Medicine and a Medical Research Council Skills Development Fellow in the School of Engineering and Materials Science, Queen Mary University of London. Caroline has a background in both mathematics and biomedical engineering, with an MMath in Mathematics from the University of Oxford, and an MRes in Biomedical Research from Imperial College London. Caroline received her PhD degree in signal processing of cardiac arrhythmia data from the Department of Bioengineering and National Heart and Lung Institute, Imperial College London (2011-2015). She then worked in the computational modelling team at Liryc, University of Bordeaux, funded by a Fondation Lefoulon Delalande fellowship (2015-2017). Recently, she was an MRC research fellow at King’s College London working in the Cardiac Electro-Mechanics Research Group (2017-2021).
Caroline’s research interests are in developing engineering methodologies for investigating the mechanisms that underlie cardiac arrhythmias and utilising this insight to develop personalised treatment approaches. We use a combination of signal processing, image processing, machine learning and computational modelling techniques to develop novel methodologies for investigating cardiac arrhythmia mechanisms from clinical imaging data and electrical recordings. For our research, we work closely with clinical, basic science and industrial collaborators.
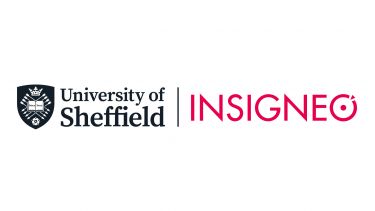