Dr Mohammad Eissa
School of Electrical and Electronic Engineering
Lecturer in Digital Electronics
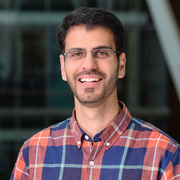
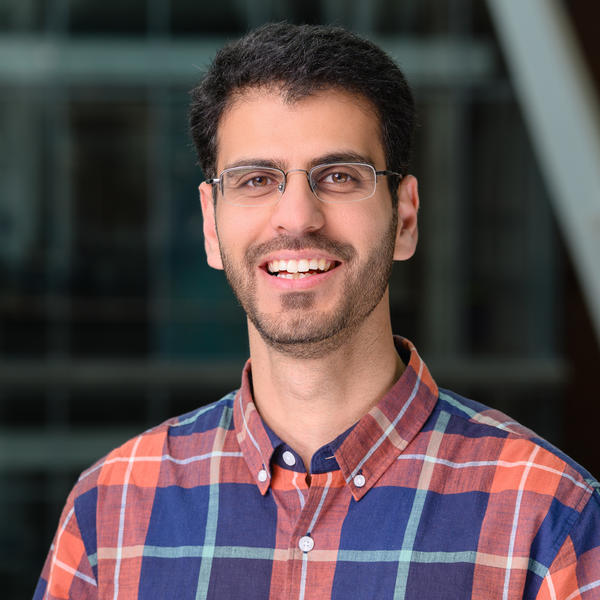
- Profile
-
I earned a Bachelor's degree in Computer Engineering followed by an MSc in Data Communication Engineering at the University of Sheffield. Then, I pursued a PhD with a focus on translational digital engineering in chronic conditions to sustain behaviour change.
I have had the privilege of holding several postdoctoral research associate roles in the Electronic and Electrical Engineering Department at the University of Sheffield. In these roles, I focused on researching novel statistical, machine learning and AI models and biomarkers. I've also ventured into digital healthcare technology with a mission to make it more inclusive and efficient. I've had the opportunity to collaborate closely with the Royal College of Arts, combining design and technology to create user-friendly healthcare solutions. My emphasis in this endeavour has been on highlighting the potential of digital technologies to enhance care, especially for vulnerable populations.
Furthermore, I've been an honorary researcher at Sheffield Teaching Hospitals. In this capacity, I was involved in analysing various aspects of diabetes care in clinical settings. This has encompassed examining the utilisation of insulin pumps and addressing the impact of the COVID-19 pandemic on people with diabetes. I've also been involved in the DAFNEplus randomised clinical trial in harnessing the power of digital technologies to enhance patient-centred care.
As I look ahead in my career, my focus remains on extending industrial partnerships in academia and further research, particularly in the healthcare technology and data-driven solutions domain. I'm passionate about System on Chip (SoC), hardware, and embedded implementations. Machine learning and AI hold a special place in my research interests due to their potential to significantly enhance decision-making and predictions in healthcare. Moreover, I actively work to make research practical and directly applicable in the real world.
- Qualifications
-
PhD (Electronic and Electrical Engineering), University of Sheffield 2023
- Research interests
-
- Machine learning and AI
- Digital healthcare technology
- IoT applications
- System on Chip (SoC)
- Hardware design
- Publications
-
Journal articles
- Automatic inference of hypoglycemia causes in type 1 diabetes: a feasibility study. Frontiers in Clinical Diabetes and Healthcare, 4.
- COVID-19 mortality risk assessments for individuals with and without diabetes mellitus: Machine learning models integrated with interpretation framework. Computers in Biology and Medicine, 105361-105361.
- Blood Glucose Level Prediction: Advanced Deep-Ensemble Learning Approach.. IEEE J Biomed Health Inform, PP.
- 6G wireless communications networks: a comprehensive survey. IEEE Access.
- Protocol for a cluster randomised controlled trial of the DAFNEplus (Dose Adjustment for Normal Eating) intervention compared with 5x1 DAFNE : a lifelong approach to promote effective self-management in adults with type 1 diabetes. BMJ Open, 11(1). View this article in WRRO
- Intelligent Data-Driven Model for Diabetes Diurnal Patterns Analysis. IEEE Journal of Biomedical and Health Informatics, 24(10), 2984-2992.
- A deep neural network application for improved prediction of HbA1c in type 1 diabetes. IEEE Journal of Biomedical and Health Informatics. View this article in WRRO
- Classification before regression for improving the accuracy of glucose quantification using absorption spectroscopy. Talanta. View this article in WRRO
- Corrigendum: Automatic inference of hypoglycemia causes in type 1 diabetes: a feasibility study. Frontiers in Clinical Diabetes and Healthcare, 4.
- Analysis of real‐world capillary blood glucose data to help reduce
HbA1c and hypoglycaemia in type 1 diabetes: evidence in favour of using percentage of readings in target and coefficient of variation. Diabetic Medicine.
- Automatic inference of hypoglycemia causes in type 1 diabetes: a feasibility study. Frontiers in Clinical Diabetes and Healthcare, 4.
- Teaching activities
-
- EEE125
- EEE232
- EEE6225