Professor George Panoutsos
MSc, PhD, FHEA
School of Electrical and Electronic Engineering
Head of School
Professor of Computational Intelligence
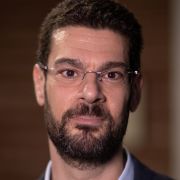
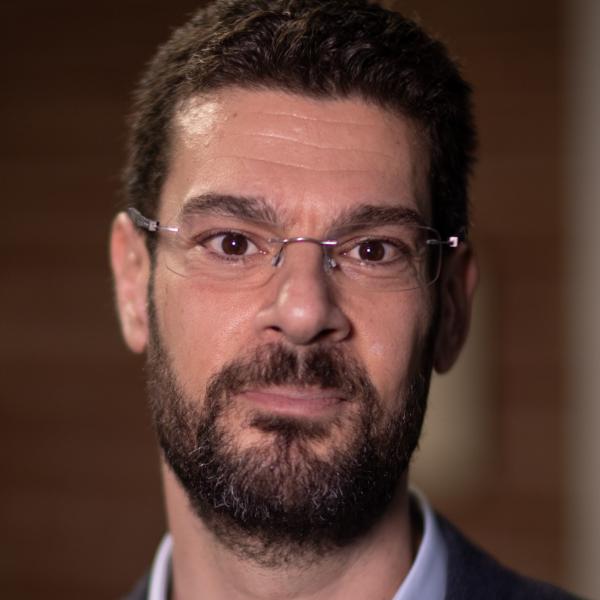
+44 114 222 5130
Full contact details
School of Electrical and Electronic Engineering
Amy Johnson Building
Portobello Street
Sheffield
S1 3JD
- Profile
-
George Panoutsos received his PhD degree in automatic control and systems engineering from the University of Sheffield, Sheffield, U.K, in 2007. He joined the Department of Automatic Control and Systems Engineering (University of Sheffield, UK) as a Lecturer in 2010, and promoted to Professor of Computational Intelligence in 2019.
George has a research grant portfolio of over £3M from the UK EPSRC, Innovate UK, DSTL, EU Horizon 2020 and direct industry funding, as well as over 100 research publications in theoretical as well as applied contributions in the areas of computational intelligence, data-driven modelling, optimisation, control, and decision support systems.
In terms of applied research, the majority of his work is on advanced manufacturing systems, as well as healthcare applications, while also currently exploring research applications in energy and infrastructure.
- Research interests
-
My research focuses on explainable and trustworthy machine learning (ML). Explainability is multifaceted in this context; I work on mathematical and computational methods in Computational Intelligence (CI) that enable enhanced understanding and transparent information use for neural networks, visual and numerical performance measures for many-objective optimisation algorithms, as well as linguistic interpretations of models, and safe control systems. Explainability and trustworthiness are key barriers in using machine learning in a range of critical applications, e.g. in engineering, and healthcare. A multitude of research questions still need to be addressed, for example how neural network - based systems learn and perform when information/data is imperfect, how can we exploit prior knowledge for enhanced learning, and how can we develop performance metrics that will allow us to understand the optimisation of systems at scale.
Towards formulating research questions in machine learning, I often use challenge-driven research e.g. in manufacturing, healthcare, as case studies. This way, applications drive the research questions, towards maximising impact. I also use explainable machine learning for translational research and to create innovation to address global challenges (e.g. sustainability, energy). The advanced monitoring, optimisation and control of manufacturing processes is such an example, where ML-based methods can be used to reduce material waste, and minimise energy use.
I welcome PhD applications in topics that fall under Computational Intelligence, in particular when these are concerned with explainable machine learning. Examples of recent PhD projects include, physics-guided neural networks, physics-guided generative models, new performance metrics for decomposition-based many-objective optimisation, information theoretic explainability in neural networks, safe reinforcement learning, and linguistic interpretations of Convolutional Neural Networks.
Recent research awards and projects
-
2019-2021, Innovate UK, 'VULCAN' Data efficient process-part monitoring and control for Electron Beam Melting using Machine Learning (PI, £268k)
-
2019, UoS ACSE pump-prime fund, A phenotypical knowledge-based image classifier for identification of novel anti-metastasis drugs (PI, £2k)
-
2019-2021 EPSRC, Using Machine learning to enable feedback controlled manufacture of self-assembled patterned materials (co-I £250k)
- 2019-2022, Aerospace Technology Institute, DAM, Developing Design for Additive Manufacturing, (co-I, ACSE project £186k)
- 2019-2022, Aerospace Technology Institute, AIRLIFT, Additive IndustRiaLIsation FuTure Technology, (co-I, ACSE project £140k)
- 2016-2022 EPSRC MAPP Hub, Future Powder Manufacturing Hub (co-I £10M)
- 2018-2020 EU H2020, INTEGRADDE (co-I £12.7M)
- 2017-2019 Innovate UK, MIRIAM - Machine Intelligence for Radically Improved Additive Manufacturing (co-I £666k)
- 2016 -2018 Innovate UK, TACDAM, Tailorable & Adaptive Connected Digital Additive Manufacturing (academic PI £1M )
- 2018-2020 TWI Ltd, Phased array NDT in Stir Welding: Interpretable machine learning and process monitoring (PI £42k)
- 2015-2017 EU H2020, Factories of The Future - 01: Process Optimisation of Manufacturing Assets, COMBILASER, (co-I and academic lead, £3.48M)
- 2014-2016 TSB, Sustained Process Excellence through Embedding of Analytics and Knowledge Management into Process Chains, Academic (PI, total project cost £441k)
- 2013-2014 METRC Innovation Award, Online and real-time condition monitoring of Friction Stir Welding, (PI £10k)
- 2012 EPSRC/Sheffield University, Model-based performance evaluation for critical manufacturing processes (PI £61k)
- 2012-2014 TWI Ltd. Yorkshire, UK, Automated Systems for Intelligent Stir Tracking and Optimisation (PI £29k)
- 2010-2013 TWI Ltd. Cambridge, UK, Multiscale model-based search for optimal Process Operating Windows in Friction Stir Welding (PI £6k)
-
- Publications
-
Journal articles
- Information-theoretic sensor placement for large sewer networks. Water Research, 122718-122718.
- Real-Time Object Detection and Robotic Manipulation for Agriculture Using a YOLO-Based Learning Approach. 2024 IEEE International Conference on Industrial Technology (ICIT).
- Synchrotron imaging derived relationship between process parameters and build quality for directed energy deposition additively manufactured IN718. Additive Manufacturing Letters, 6.
- Stability in Reinforcement Learning Process Control for Additive Manufacturing. IFAC-PapersOnLine, 56(2), 4719-4724.
- An evolving feature weighting framework for radial basis function neural network models. Expert Systems.
- Hyaluronan nanoscale clustering and Hyaluronan synthase 2 expression are linked to the invasion of child fibroblasts and infantile fibrosarcoma in vitro and in vivo. Scientific Reports, 12, 1-10. View this article in WRRO
- Application of transfer learning for the prediction of blast impulse. International Journal of Protective Structures. View this article in WRRO
- Structure optimization of prior-knowledge-guided neural networks. Neurocomputing, 491, 464-488. View this article in WRRO
- Physics-informed regularisation procedure in neural networks: An application in blast protection engineering. International Journal of Protective Structures.
- Data-driven granular computing systems and applications. Granular Computing. View this article in WRRO
- A data-driven approach for predicting printability in metal additive manufacturing processes. Journal of Intelligent Manufacturing. View this article in WRRO
- A Multilayer Interval Type-2 Fuzzy Extreme Learning Machine for the recognition of walking activities and gait events using wearable sensors. Neurocomputing, 389, 42-55. View this article in WRRO
- Transient thermography for flaw detection in friction stir welding : a machine learning approach. IEEE Transactions on Industrial Informatics. View this article in WRRO
- Methods for rapid pore classification in metal additive manufacturing. JOM Journal of the Minerals, Metals and Materials Society. View this article in WRRO
- Long-term Learning for Type-2 Neural-Fuzzy Systems. Fuzzy Sets and Systems, 368, 59-81. View this article in WRRO
- Using Multiple-Feature-Spaces-Based Deep Learning for Tool Condition Monitoring in Ultraprecision Manufacturing. IEEE Transactions on Industrial Electronics, 66(5), 3794-3803. View this article in WRRO
- General Type-2 Radial Basis Function Neural Network: A Data-Driven Fuzzy Model. IEEE Transactions on Fuzzy Systems, 27(2), 333-347. View this article in WRRO
- Project SunbYte: solar astronomy on a budget. Astronomy and Geophysics, 58(2), 2.24-2.25. View this article in WRRO
- A real-time quality monitoring framework for steel friction stir welding using computational intelligence. Journal of Manufacturing Processes, 20(P1), 137-148.
- Multiobjective optimal design of friction stir welding considering quality and cost issues. Science and Technology of Welding and Joining, 20(7), 607-615. View this article in WRRO
- High Dimensionality and Scaling-up Performance of RBF Models with Application to Healthcare Informatics. International Journal of Machine Learning and Computing, 5(1), 62-67.
- Interval Type-2 Radial Basis Function Neural Network: A Modeling Framework. IEEE Transactions on Fuzzy Systems, 23(2), 457-473.
- Granular computing neural-fuzzy modelling: A neutrosophic approach. APPLIED SOFT COMPUTING, 13(9), 4010-4021.
- Towards a Patient-Specific Model of Lung Volume Using Absolute Electrical Impedance Tomography (aEIT), 273, 191.
- Knowledge discovery for friction stir welding via data driven approaches part 1 - Correlation analyses of internal process variables and weld quality. Science and Technology of Welding and Joining, 17(8), 672-680.
- Knowledge discovery for friction stir welding via data driven approaches Part 2 - Multiobjective modelling using fuzzy rule based systems. Science and Technology of Welding and Joining, 17(8), 681-693.
- Towards a Patient-Specific Model of Lung Volume Using Absolute Electrical Impedance Tomography (aEIT). Communications in Computer and Information Science, 273, 191-204.
- Development of a parsimonious GA-NN ensemble model with a case study for Charpy impact energy prediction. Advances in Engineering Software, 42(7), 435-443.
- Modeling and Optimal Design of Machining-Induced Residual Stresses in Aluminium Alloys Using a Fast Hierarchical Multiobjective Optimization Algorithm. Materials and Manufacturing Processes, 26(3), 508-520.
- Intelligent model-based advisory system for the management of ventilated intensive care patients. Part II: Advisory system design and evaluation. Computer Methods and Programs in Biomedicine, 99(2), 208-217.
- Real-time adaptive automation system based on identification of operator functional state in simulated process control operations. IEEE Transactions on Systems, Man, and Cybernetics Part A:Systems and Humans, 40(2), 251-262.
- A neural-fuzzy modelling framework based on granular computing: Concepts and applications. Fuzzy Sets and Systems, 161(21), 2808-2830.
- Absolute electrical impedance tomography (aEIT) guided ventilation therapy in critical care patients: Simulations and future trends. IEEE Transactions on Information Technology in Biomedicine, 14(3), 641-649. View this article in WRRO
- Intelligent model-based advisory system for the management of ventilated intensive care patients: Hybrid blood gas patient model. Computer Methods and Programs in Biomedicine, 99(2), 195-207.
- "right-first-time" production: A reality or a myth?. Materials and Manufacturing Processes, 24(1), 78-82.
- Deep multi-metric training: the need of multi-metric curve evaluation to avoid weak learning. Neural Computing and Applications.
- TabNet: Locally Interpretable Estimation and Prediction for Advanced Proton Exchange Membrane Fuel Cell Health Management. Electronics, 13(7), 1358-1358.
- In-situ porosity prediction in metal powder bed fusion additive manufacturing using spectral emissions: a prior-guided machine learning approach. Journal of Intelligent Manufacturing.
- Predicting specific impulse distributions for spherical explosives in the extreme near-field using a Gaussian function. International Journal of Protective Structures.
Chapters
- A Fuzzy Logic-Based Framework for Statistical Process Control in Additive Manufacturing, Advances in Intelligent Systems and Computing (pp. 61-72). Springer Nature Switzerland
- Improving the Explainability of Multi-criteria Decision-Making Using Neutrosophic Logic, Advances in Intelligent Systems and Computing (pp. 551-562). Springer Nature Switzerland
- Overlapping Granular Clustering: Application in Fuzzy Rule-Based Classification, Lecture Notes in Networks and Systems (pp. 84-93). Springer Nature Switzerland
- Interpretable Convolutional Neural Networks Using a Rule-Based Framework for Classification, Intelligent Systems: Theory, Research and Innovation in Applications (pp. 1-24). Springer International Publishing
- Data-Driven Interval Type-2 Fuzzy Modelling for the Classification of Imbalanced Data, Studies in Systems, Decision and Control (pp. 37-51).
- An incremental learning structure using granular computing and model fusion with application to materials processing (pp. 139-153).
Conference proceedings papers
- Output Tracking for Uncertain Time-Delay Systems via Robust Reinforcement Learning Control. 2024 43rd Chinese Control Conference (CCC), Vol. 104 (pp 2219-2226), 28 July 2024 - 31 July 2024.
- A Reinforcement Learning-Based Approach for Optimal Output Tracking in Uncertain Nonlinear Systems with Mismatched Disturbances. 2024 UKACC 14th International Conference on Control (CONTROL), 10 April 2024 - 12 April 2024.
- Reinforcement Learning-Based Output Stabilization Control for Nonlinear Systems With Generalized Disturbances. 2024 IEEE International Conference on Industrial Technology (ICIT), 25 March 2024 - 27 March 2024.
- Reinforcement Learning for Multiple-Input Multiple-Output Control in Metal Additive Manufacturing. 2023 IEEE International Conference on Networking, Sensing and Control (ICNSC), 25 October 2023 - 27 October 2023.
- High Dimensional Many Objective Optimisation through Diverse Creation and Categorisation of Reference Vectors. Proceedings of the Companion Conference on Genetic and Evolutionary Computation
- Exploiting Laguerre polynomials and steady-state estimates to facilitate tuning of PFC. 2022 European Control Conference (ECC), 12 July 2022 - 15 July 2022. View this article in WRRO
- A novel approach to PFC for nonlinear systems. European Journal of Control (pp 100668-100668) View this article in WRRO
- On-The-Fly CNC interpolation using frequency-domain FFT-based filtering. Procedia CIRP, Vol. 107 (pp 1571-1576). Lugano, Switzerland, 29 June 2022 - 1 July 2022.
- Predictive Functional Control for Difficult Second-Order Dynamics with a Simple Pre-compensation Strategy. 2022 UKACC 13th International Conference on Control (CONTROL), Vol. 00 (pp 12-17) View this article in WRRO
- Predictive Functional Control for Difficult Dynamic Processes with a Simplified Tuning Mechanism. 2022 UKACC 13th International Conference on Control (CONTROL), Vol. 00 (pp 130-135) View this article in WRRO
- Fuzzy Multi-Criteria Decision-Making: Example of an Explainable Classification Framework. UKCI 2021: Advances in Computational Intelligence Systems, Vol. 1409 (pp 15-26). Aberystwyth, United Kingdom, 8 September 2021 - 10 September 2021.
- An Evolving Feature Weighting Framework for Granular Fuzzy Logic Models. UKCI 2021: Advances in Computational Intelligence Systems, Vol. 1409 (pp 3-14). Aberystwyth, UK, 8 September 2021 - 10 September 2021.
- View this article in WRRO
- Mental Workload Estimation using Wireless EEG Signals. Proceedings of the 14th International Joint Conference on Biomedical Engineering Systems and Technologies, 11 February 2021 - 13 February 2021.
- Multi-criteria decision making using Fuzzy Logic and ATOVIC with application to manufacturing. 2020 IEEE International Conference on Fuzzy Systems (FUZZ-IEEE). Glasgow, UK, 19 July 2020 - 24 July 2020.
- A New Method for Generating and Indexing Reference Points in Many Objective Optimisation. 2020 IEEE Congress on Evolutionary Computation (CEC), 19 July 2020 - 24 July 2020.
- Interpretable Machine Learning: Convolutional Neural Networks with RBF Fuzzy Logic Classification Rules. Proceedings of the 2018 International Conference on Intelligent Systems (pp 448-454), 25 September 2018 - 27 September 2018. View this article in WRRO
- Evolutionary Extreme Learning Machine for the Interval Type-2 Radial
Basis Function Neural Network: A Fuzzy Modelling Approach. 2018 IEEE International Conference on Fuzzy Systems (FUZZ-IEEE), 8 July 2018 - 13 July 2018. View this article in WRRO
- A combined Adaptive Neuro-Fuzzy and Bayesian strategy for recognition and prediction of gait events using wearable sensors. IEEE International Conference on Fuzzy Systems View this article in WRRO
- An ensemble data-driven fuzzy network for laser welding quality prediction. 2017 IEEE International Conference on Fuzzy Systems, 9 July 2017 - 12 July 2017. View this article in WRRO
- Perpetual Learning Framework based on Type-2 Fuzzy Logic System for a Complex Manufacturing Process. IFAC-PapersOnLine, Vol. 49(20) (pp 143-148) View this article in WRRO
- Iterative Information Granulation for Novelty Detection in Complex Datasets. 2016 IEEE International Conference on Fuzzy Systems (FUZZ-IEEE), 24 July 2016 - 29 July 2016. View this article in WRRO
- A Data-driven fuzzy modelling framework for the classification of imbalanced data. 2016 IEEE 8th International Conference on Intelligent Systems (IS), 4 September 2016 - 6 September 2016.
- Model-Based Feature Selection Based on Radial Basis Functions and Information Measures. 2016 IEEE International Conference on Fuzzy Systems (FUZZ-IEEE), 24 July 2016 - 29 July 2016. View this article in WRRO
- A Shannon entropy-based conflict measure for enhancing granular computing-based information processing. 2014 IEEE International Conference on Granular Computing (GrC), 22 October 2014 - 24 October 2014.
- Suggestive therapeutic pathways using hyper-heuristics. IFAC Proceedings Volumes (IFAC-PapersOnline), Vol. 45(18) (pp 397-402)
- A multi-objective particle swarm optimization algorithm with a dynamic hypercube archive, mutation and population competition. 2012 IEEE Congress on Evolutionary Computation, CEC 2012
- Systems-modelling of compact tension energy in high strength pipeline steel. IFAC Proceedings Volumes (IFAC-PapersOnline), Vol. 44(1 PART 1) (pp 12126-12131)
- An integrated system approach for solving multi-objective scheduling problems and advanced control of an experimental hot-rolling mill. IFAC Proceedings Volumes (IFAC-PapersOnline), Vol. 44(1 PART 1) (pp 12084-12089)
- Confidence interval assessment for Charpy impact energy predictions - A Gaussian Mixture Model (GMM)-based approach. IFAC Proceedings Volumes (IFAC-PapersOnline), Vol. 44(1 PART 1) (pp 11738-11743)
- Model fusion via a master fuzzy system with special application to engineering materials. IFAC Proceedings Volumes (IFAC-PapersOnline), Vol. 44(1 PART 1) (pp 11732-11737)
- Multiple characterisation modelling of friction stir welding using a genetic multi-objective data-driven fuzzy modelling approach. IEEE International Conference on Fuzzy Systems (pp 2288-2295)
- Adaptive neural-fuzzy inference system for classification of rail quality data with bootstrapping-based over-sampling. IEEE International Conference on Fuzzy Systems (pp 2205-2212)
- A generic framework for enhancing the interpretability of granular computing-based information. 2010 IEEE International Conference on Intelligent Systems, IS 2010 - Proceedings (pp 19-24)
- The assessment of Heart Rate Variability (HRV) and Task Load Index (TLI) as physiological markers for physical stress. IFMBE Proceedings, Vol. 25(13) (pp 146-149)
- Goal-Directed Therapy for General ICU Patients Using Aggregated Multi-Objective Optimisation. IFAC Proceedings Volumes, Vol. 41(2) (pp 9063-9068)
- Goal-directed therapy for general ICU patients using aggregated multi-objective optimisation. IFAC Proceedings Volumes (IFAC-PapersOnline), Vol. 17(1 PART 1)
- Modelling imprecise and scattered multidimensional data using granular data compression and multiple granularity modelling. 2008 IEEE International Conference on Granular Computing, GRC 2008 (pp 512-517)
- An incremental learning structure using granular computing and model fusion with application to materials processing. IEEE Intelligent Systems (pp 367-372)
- View this article in WRRO
- View this article in WRRO
- View this article in WRRO
- View this article in WRRO
Patents
Preprints
- The relative contributions of subjective and musical factors in music for sleep.
- A novel framework employing deep multi-attention channels network for the autonomous detection of metastasizing cells through fluorescence microscopy, arXiv.
- Hyaluronan nanoscale clustering and Hyaluronan synthase 2 expression are linked to the invasion of child fibroblasts and infantile fibrosarcoma in vitro and in vivo, Research Square Platform LLC.
- Mental Workload Estimation Using Wireless EEG Signals, Cold Spring Harbor Laboratory.
- Information-theoretic sensor placement for large sewer networks. Water Research, 122718-122718.
- Grants
-
Current Grants
- Diode area melting - a novel re-configurable multi-laser approach for efficient additive manufacturing with enhanced thermal process control, RCUK, 01/06/2022 - 30/11/2024, £629,880, as Co-PI
- NanoMan: Self-Optimising Nanoscale Manufacturing Platforms for Achieving Multiscale Precision, RCUK, 13/01/2022 - 12/01/2025, £1,432,279, as Co-PI
- Responsive Manufacturing of High Value Thin to Thick Films, RCUK, 01/09/2021 - 31/08/2024, £2,025,997, as Co-PI
- DAM: Developing Design for Additive Manufacturing, Innovate UK, 01/12/2018 - 30/11/2022, £930,941, as Co-PI
- Integradde, EU H2020, 01/10/2018 - 31/03/2023, £694,628, as Co-PI
- MAPP: EPSRC Future Manufacturing Hub in Manufacture using Advanced Powder Processes, RCUK, 01/10/2016 - 30/09/2023, £6,776,372, as Co-PI
Previous Grants
- AIRLIFT: Additive IndustrRiaLIsation for Future Technology), Innovate UK, 01/12/2018 - 30/11/2023, £545,174, as Co-PI
- Machine Learning digital twin for defect-free additive manufacturing, Research England, 01/02/2022 - 30/06/2022, £29,551, as Co-PI
- Materials 4.0, RCUK, 01/01/2022 - 31/03/2022, £54,647, as PI
- CMAC Feasibility Study, RCUK, 01/10/2021 - 30/09/2022, £59,982, as PI
- VULCAN, Innovate UK, 01/11/2019 - 31/01/2022, £334,563, as PI
- Using Machine learning to enable feedback controlled manufacture of self-assembled patterned materials, RCUK, 30/09/2019 - 28/03/2022, £252,938, as Co-PI
- TACDAM: Tailorable & Adaptive Connected Digital Additive Manufacturing, RCUK, 01/01/2017 - 31/12/2018, £221,611, as PI
- MIRIAM: Machine Intelligence for Radically Improved Additive Manufacturing, Innovate UK, 01/10/2017 - 31/03/2019, £261,312, as Co-PI
- Integrated machine-part multi-objective optimisation for powder manufacturing, RCUK, 01/11/2016 - 3103/2017, £40,000, as PI
- Teaching activities
-
- ACS6402, Industry Training Programme in Advanced Manufacturing (module leader)
- ACS6403, Industry Training Programme in Computational Intelligence (module leader)