Improving Prediction of Low Adhesion Friction using Machine Learning Approaches
The University of Sheffield in conjunction with the Railway Safety and Standards Board (RSSB) are working to improve the understanding of wheel/rail interface low adhesion friction prediction using machine learning approaches.
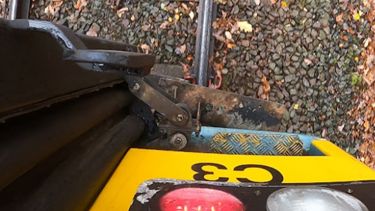
Low adhesion between the rail tracks and train wheels has been identified as one of the causes of the delays on rail lines, consequently incurring high cost. Common causes of low adhesion have been identified to be decomposed and crushed leaves on the railhead and “wet-rail” phenomenon.
This project is directed at understanding the causes and mechanisms of low adhesion and using the knowledge to further develop and validate an adhesion forecasting tool. The proposed tool is based on machine learning and neural networks that have been trained to link railhead images (see image below) and environmental data to corresponding friction levels on the rail head and aid real-time adhesion level prediction.
There is currently no validated real-time adhesion prediction tool based on lineside information and this project aims to fill this research gap. This will improve safety and reliability of the railway industry.
People involved
Morinoye O. Folorunso