Joshua Stutter
School of History, Philosophy and Digital Humanities
Teaching Associate in Digital Humanities
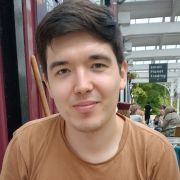
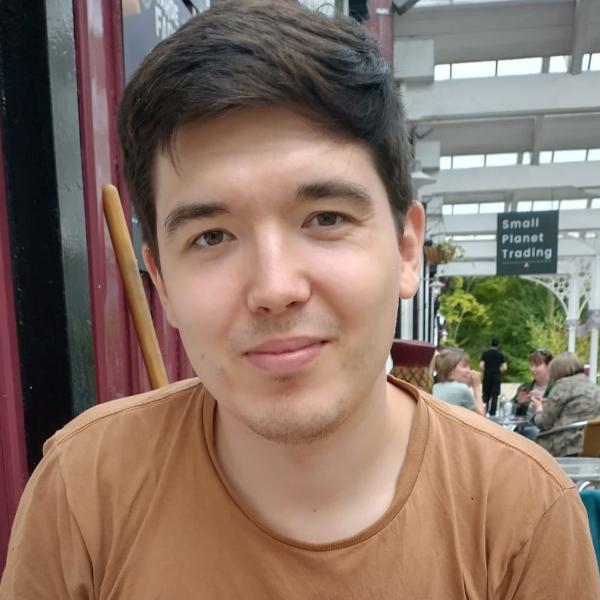
- Profile
-
Originally from Manchester, I studied music at the University of Cambridge and my PhD at the University of Glasgow. I am a singer, conductor, accompanist, editor, typesetter, and teacher, but primarily a researcher into the ways in which digital methods can be applied to (early) music, the novel problems that music poses for digital encoding and computational analysis, and the creation of large-scale corpora and tools for answering musicological questions. I am founder of early music ensemble iuchair, through whom much of my research is brought to life, and continue to work on the Clausula Archive of the Notre Dame Repertory (CANDR) project.
- Research interests
-
My recent research revolves around three core areas:
- Musical reuse in the medieval period: I examine the concept of the clausula in thirteenth-century music, exploring its significance as a medieval polyphonic element as well as its broader implications as symbol of the untold musical reuse in medieval music. I employ digital and Big Data methods to explore this, developing models and frameworks for polyphonic corpus analysis.
- Music historiography: Many terms that are commonly used are not defined exactly, or are defined variously over time. In the study of medieval music, many of these terms are Latin loanwords or neologisms. I am interested in how these terms interact in academic literature with the scholarly community, and how these terms have been invented or moulded to fit certain historical narratives.
- Digital methods in medieval music: I tackle the task of modelling ambiguous music in the computer, acknowledging the complexities that arise from music’s diverse forms, media, and the field’s methodological infancy. I research the creation of novel ontologies and data structures for representing music, addressing the transformation of these structures into vector representations for machine learning. I aim to understand and mitigate encoding biases, while also developing new music encoding models and machine learning methodologies.